Responses (0)
Welcome to the LLM model that's been absolutely everywhere on the Internet and news headlines in recent days – DeepSeek-R1! In this article, we take a comprehensive look at this new, industry-disrupting LLM. We'll investigate if it’s truly deserving of all the noise around it, or if there's something (i.e. censorship and GPT-4 references) more sinister going on beneath the buzz.
So, brew some tea and settle in, because this is going to be an interesting ride.
We're going to cover:
A short historical introduction to DeepSeek.
DeepSeek's impact and general overview.
Benchmarks and performance.
Pros and cons.
Technical Overview.
Chain of Thought Reasoning
Model Distillation for Accessibility
Reinforcement Learning for Self Improvement
Efficiency and cost
Moving from Powerful GPUs to VRAM: A Paradigm Shift
Math behind model training
Human involvement in model training
Evolution from From R1-Zero to R1
Advancement of Inference and Autonomous Agents
Impact on the future market.
Open source and how it's catching up.
How to install DeepSeek locally.
So, feel free to jump into any section that interests you. But I recommend taking your time and going through all of it to get a clear picture of everything.
A Short Historical Introduction#
Let's clarify some things first. DeepSeek is the name of a small Chinese AI firm funded by a local “High-Flyer” hedge fund, whereas DeepSeek-R1 is the name of their state-of-the-art model. Interestingly, DeepSeek-R1 was launched a week ago, nominally just as a side project for the company. But where did this company come from? To some, it might seem like it appeared out of nowhere.
DeepSeek was founded in May 2023 by Liang Wenfeng in Hangzhou, China. Even back then, it was starting to emerge as a disruptive force in AI. The company's speciality is open-source large language models (LLMs), with their main focus being on research, with no open plans for commercialization.
Their first open-source model series (DeepSeek-Coder) was released in November 2023, followed by DeepSeek-LLM later that month, competing directly with global leaders like OpenAI's ChatGPT. The models gained recognition for their performance and affordability. Since then, they have continued to release new models. Their latest model includes two variations. In December 2024, DeepSeek-V3 (non-reasoning) model was launched. And in January 2025, DeepSeek-R1 (with reasoning, more performant) was launched. DeepSeek-R1 surpassed ChatGPT-01 in quite a few metrics, and quickly rose to become the most downloaded iOS app in the U.S., while at the same time crushing the market value of many U.S. companies involved in the AI industry.
But why has such breakthrough technology emerged in China, you might ask? The driving force, ironically, lies in U.S. chip export sanctions imposed on China. Despite stockpiling around 10,000 Nvidia A100 GPUs before the sanctions, Liang (co-founder) and his team were clearly facing hardware limitations compared to giants like OpenAI. These constraints forced DeepSeek to rethink its approach to building LLMs, prioritizing efficiency and cost-effectiveness in both training and deployment.
And what has made DeepSeek-R1 so impactful? In short:
Reduced Capital Expenses (CapEx): The model dramatically lowers the capital expenditure (CapEx) required for AI deployment.
Significant reductions in the costs associated with running AI inferences.
Incorporates advanced prompt engineering techniques, which, while slower, often produce superior results.
In summary, DeepSeek's strategic focus on efficiency, coupled with China's unique technological landscape, has enabled this small firm to make a significant impact on the global AI industry. But more about that later.
DeepSeek's Impact and General Overview #
At the time of writing, $1.2 trillion has been wiped off the US stock market in the span of just a couple of days, $600 billion of which belongs to Nvidia.

But why? In the past, if we wanted to make a model larger and better, the main approach used to be just throw more horsepower at it. Of course, tech giants improved the efficiency of their models along the way. But those improvements, substantial as they may be, were not groundbreaking. Thus, hardware requirements quickly became the major bottleneck for training and running better models.
And what is the main hardware used for LLMs? As we all know – it’s GPUs, where Nvidia is dominating the market.
But DeepSeek-R1 changed the game, delivering a comprehensive LLM model with a much lower hardware footprint and cost, making everyone realize that the overreliance on raw GPU power might end really soon. Thus, all the Big Tech companies related to AI (OpenAI, Google, Meta, Nvidia) are starting to face pressure from low-cost models that are challenging their closed, expensive systems.
But is it really so efficient that it should disrupt the whole market? Let's take a closer look.
Let’s start with the fact that training top AI models is insanely expensive right now. OpenAI, Anthropic, etc., spend $100M+ just on compute alone. Additionally, they need massive data centers with thousands of super-expensive GPUs, which might cost up to $40K each, basically requiring power plant-like infrastructure to produce these cutting-edge models.
And what about DeepSeek? It trained its model for around $5M (1/20 of the price), requiring less infrastructure, less compute power, less energy consumption, and fewer engineers. Not only that, but on top of all those benefits, their models also match or even beat GPT-4 and Claude on many tasks.
But wait, there’s more. The sweet part is that DeepSeek-R1 is not only cheaper to produce, but it also requires much less powerful hardware to run, thus being cheaper overall!
Now, if you combine all the benefits and put them into numbers – the results are mind-blowing:
Training cost: $100M → $5M
GPUs needed: 100,000 → 2,000
API costs: 95% cheaper
Able to run an instance of the model locally with top-of-the-line gaming GPUs, instead of being limited to data center hardware only
To put it into perspective, even a 2-year-old chip like the M2 Ultra can run the largest 671B model as fast as you can read. But the only caveat here is that you need three of them due to VRAM limitations. We'll explore why VRAM becomes an important factor in the following technical section.
But! There’s a small disclaimer. Let’s not forget that the reported training cost comes from DeepSeek themselves, and for now, we have no comprehensive way to independently verify or dispute it. Until third-party testing is conducted and more transparency is provided, the true cost-effectiveness of DeepSeek-R1 remains an open question. That said, even if they’ve cherry-picked the best results, it still looks like it's way ahead of any competitors.
If you want to test the model yourself, as of January 27, 2025, the chat platform is free to use but with a daily cap of 50 messages in “Deep Think” mode. This limitation makes it ideal for light usage or exploration.
What about API? It offers two models – deepseek-chat
(DeepSeek-V3, non-reasoning model) and deepseek-reasoner
(DeepSeek-R1, reasoning model) – with the following pricing structure (per 1M tokens):

Here’s their pricing page.
Benchmarks#
Now that we have covered business benefits, let's take a closer look at how DeepSeek-V3 (non-reasoning model) and DeepSeek-R1 (reasoning model) perform compared to more established competitors. We will be focusing in particular on the state-of-the-art DeepSeek-R1.
But before we start with the analysis, I want to point out an interesting quirk that I have personally encountered, as have many others, while testing the model. What happens if we ask the model to identify and name itself? Surprisingly, from time to time, it refers to itself as GPT-4! Here are a couple of examples from different people who have also noticed such behavior.


Does this mean they just scraped GPT-4 and trained their model based on it? Maybe. But also, let's not forget that the word “GPT” is really popular and widely used online. So, potentially, because of its popularity, the trained model might use it as the default answer. Who knows what the case is, but don't be surprised if you come across such an answer. Now you know.
And back to the benchmarks.
In most cases, DeepSeek-R1 competes directly with OpenAI o1 across several benchmarks, often matching or surpassing OpenAI’s o1.

Source: DeepSeek’s release paper.
Subscribe to read the full article
This article is for subscribers only and can be unlocked immediately with a \newline Pro subscription. Already have access? Log in here.
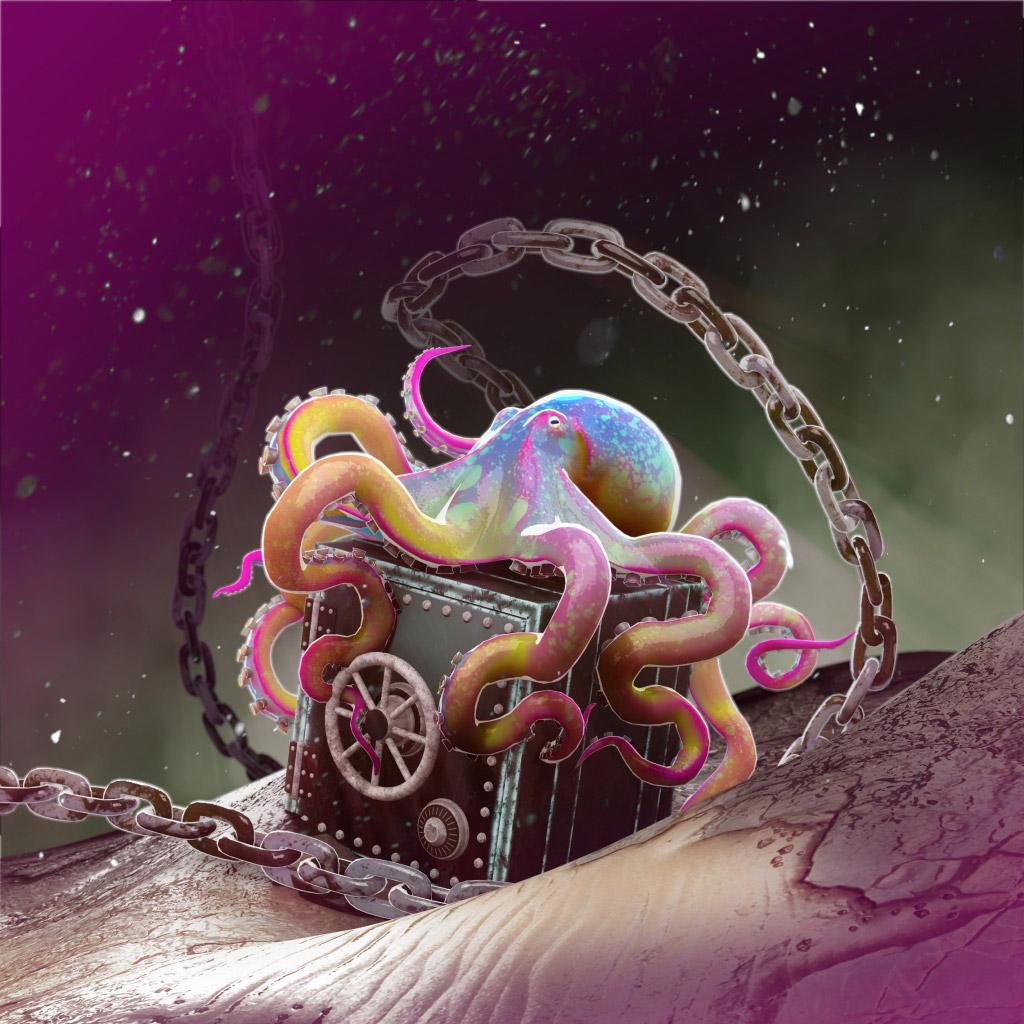